Article
Combination of the Synthetically Generated Data-sets and XAI Techniques to Provide Transparency and Robustness of the AI-powered Defence Systems

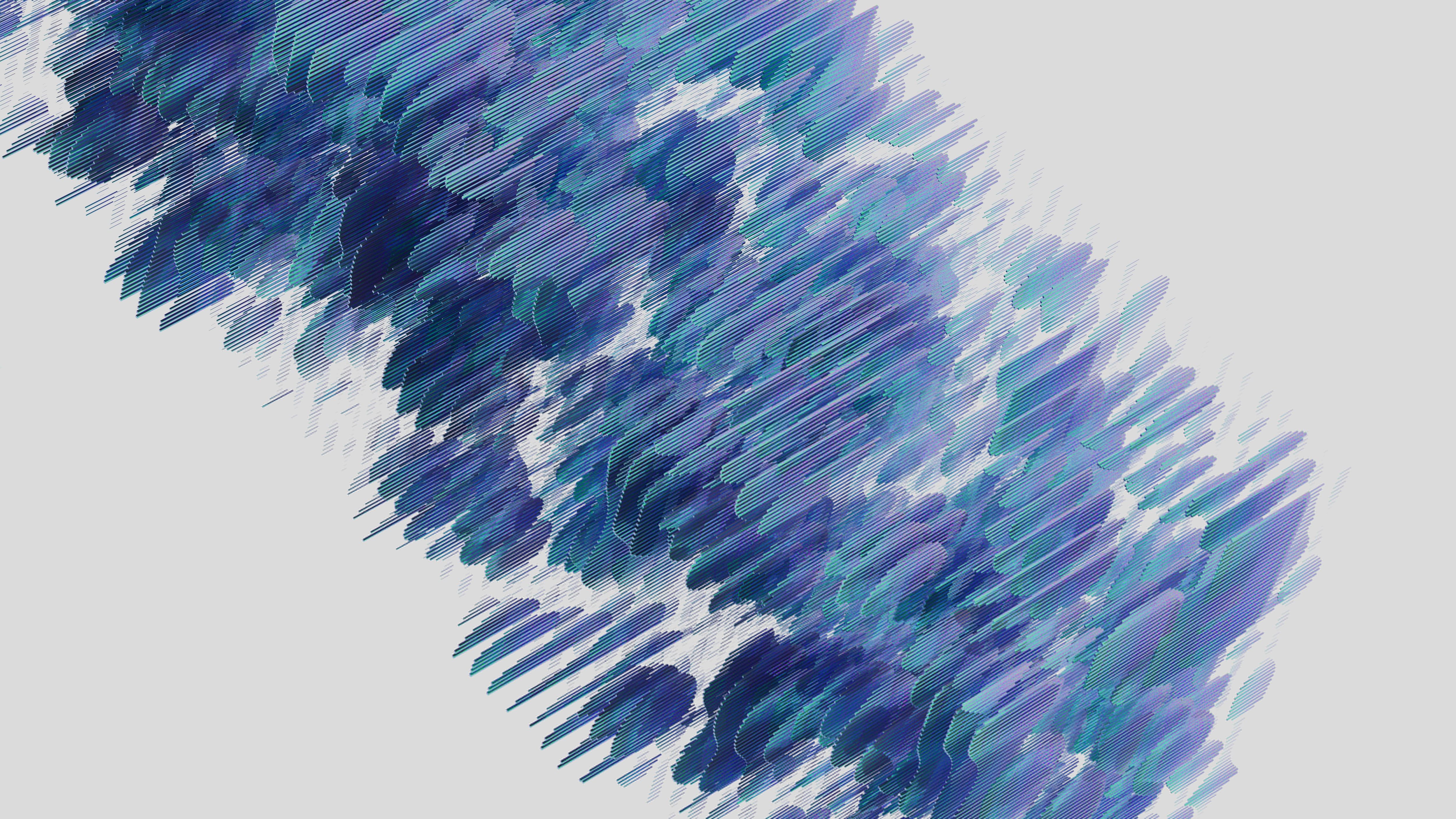
Abstract
This paper proposes utilising explainable artificial intelligence (XAI) methods and synthetically generated data sets at different levels of Artificial Intelligence (AI) development to provide robustness and transparency to AI-based defence systems. Unlike the state-of-the-art solutions, we propose using XAI methods not only as the post-hock interpreters but as the support for different levels of the development and integration of AI components. In this setting, synthetic data sets are proposed not only to overcome problems related to data scarcity or restriction accesses but also to create controlled environments that allow evaluation of the goodness of all components of the XAI model. This approach will improve the robustness of the XAI component and support compliance with ethical and law-related guidelines.
Introduction
In recent years, the application of AI has attracted much attention from academia, business, and industry. There are two main properties that distinguish success stories from those in which the potential of AI has not yet been used. The first is the synergy between AI specialists and their counterparts in the field where AI is applied. The second is the availability of sufficiently large data sets for the training and validation of AI models. Achieving the first property may be done by using currently available AI-decision interpreters to complement properly organised human-to-human cooperation. This will lead to human-on-the-loop oversight. Fulfilling the second property will face a more serious gap. Namely, defence data may be classified, be of insufficient size, or biased towards particular use-case. Synthetic data sets have been successfully used in many other areas to provide adequately balanced and sufficiently large amounts of AI training and validation. The framework described below proposes the extension of existing approaches beyond the state-of-the-art.
Proposed approach
XAI is AI with the capability to justify its decisions in terms of input data. The current XAI workflow limits the usage of explanations only on the post-hock step of the classical AI workflow. We extend the application of this step to the model building step one, providing the AI-specialist with the extra feedback explaining the failures of the model and drawbacks of the data preparation procedure. This approach would increase the robustness of the models. In addition, this action will increase the transparency of the model. This in turn allows for better supervision of compliance with law and ethics requirements. Considering the loops corresponding to the human-in-command oversight type, where AI-based system is able to predict consequences of the commands given by the human agent and explain them. In addition, AI may request additional information and provide an explanation for why this information is requested.
Conclusions
The XAI domain is a comparatively new field of research. Despite promising results, it is important to consider the drawbacks associated with successful applications of the XAI methods. The first is the absence of a widely accepted (agreed) system of notation and definitions. The second problem is the bias towards human-centring approaches. Although in first view this may seem to be the main goal of XAI, such bias limits the applicability of formal methods in evaluation of the quality of XAI.
Download the full document here:
__
This paper has been published as a response to the European Defence Agency call for industry, academia and RTO inputs on their views regarding how AI should be trusted for safe use in Defence Applications. It is conducted by Dr. Sven Nõmm, Head of AI/ML at SensusQ and Dr. Adrian Venables, Information Operations Scientific Advisor at SensusQ.